Pivotal to this advance was a Google machine-learning triumph in the ancient game of Go. Invented in China some 4,000 years ago, Go is a game of territorial control and maneuver, providing 361 points of intersection for patterns of black and white stones successively positioned in elaborate geometries across the board. The player who surrounds and captures the most territory wins. In Seoul in 2016, Lee Sedol, a 33-year-old-Korean and the 18-time human champion of Go, played against AlphaGo, a machine learning program created by Google’s DeepMind division led by Hinton and Sutskever. And Sedol lost four out of five engagements. More portentous still, in October 2017 Google’s DeepMind launched AlphaGo Zero. This version was based solely on reinforcement learning, without direct human input beyond the rules of the game. In a form of “generic adversarial program,” AlphaGo Zero vied against itself repeatedly billions of times. It became its own teacher. “Starting tabula rasa,” a paper by the developers concludes, “our new program AlphaGo Zero achieved superhuman performance, winning 100-0 against the previously published, champion-defeating AlphaGo.” The program employed two key machine learning techniques. One, invented by venerable Google guru Hinton, is “backpropagation.” Feeding back the errors, this method corrects the system by adjusting all the neural weights of its “neuron” filters. The entire network adapts until the outputs conform to a pattern of targets— such as a winning position in Go. The second breakthrough is “genetic programming,” popularized by Michigan’s John Holland, which “evolves” new techniques by competitive survival of the fittest. Using similar techniques, AI has mastered such previously intractable fields as protein folding (a 2019 Google accomplishment) and stock market trading (Renaissance Technologies, 1995-2020). In retrospect, AI skeptics like me disparage such feats as mere rote computer processing. The program can make millions of moves or “investments” or logical steps a second while its human adversary mulls fecklessly over one. This advantage should be enough to prevail in any logical competition that doesn’t have intrinsic information entropy or surprise. But none of us AI critics actually predicted such a machine success as the Go championship. In a game of logic and strategy, a machine learned how to defeat a world champion human by dint of computer pattern-recognition and feedback loops alone. No question, that’s an awesome achievement, and Sutskever was at the center of it. Thus, his plausible claim to lead the human race in intelligence. Sutskever now believes, so he told New Yorker scribe John Seabrook, that even people like himself may well soon be eclipsed in creativity, intelligence, and writing ability by a machine. “Researchers cannot disallow,” he says, “the possibility that we [in developing AI] will reach understanding, when the neural net gets as big as the brain… if you train a system which predicts the next word well enough then it ought to understand.” "The Coming 'Reboot' is Great News," says #1 Futurist Today’s Prophecy As I have explained in Life After Google and in a short book on AI soon to be forthcoming from Discovery Institute, I regard this Sutskever faith as a stupid materialist religion. As philosopher Charles Peirce showed early in the 20th century, logical systems such as mathematics or computational Boolean algebra consist of symbols and objects. Like maps and territories, they are not self-evidently linked. They require a human “interpretant” to make the connections across the inevitable epistemic gap. A game like Go is entirely a map or symbol system. No territory is involved, so it can be “won” without “understanding” or interpreting anything. Black and white stone symbols are all there is. Now he is using the same essential technology to create GTP3, which seeks to “understand” words and stories. GTP3 is the Peircean interpretant between its own symbols, which are words, and its objects, which are the fabric of mind, narrative, story, and meaning. In its effort to achieve an author’s creativity or imagination, entropy or surprisal, the GTP3 writer-interpreter makes the blunder of using randomness, or “stochastic” techniques. But randomness does not add information. It subtracts information. Randomness conveys entropy but not meaning. It resembles creativity mathematically but is actually just noise. Confusing the two is the fundamental error of prevailing computer fashions. Seabrook’s New Yorker story tells of GTP’s failure, given the entire New Yorker archives, to write a “New Yorker” story that made any sense at all. Treating words like musical notes or Go positions — symbols without objects — GPT produced an accurate simulation of language without its meaning. Thus, it generated a tintinnabulation of New Yorker sounds without deeper significance. That’s called gibberish. My Prophecy: If GPT3 could actually make a general-purpose machine learner and writer that could outperform humans, there would be little or no market for any of our other companies or prophecies. We could all retire to the beach. But Sutskever’s OpenAI is Silicon Valley religion and will probably dwindle into an enthusiasm of AI dilettantes. Regards, 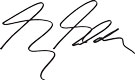 George Gilder Editor, Gilder's Daily Prophecy |
No comments:
Post a Comment